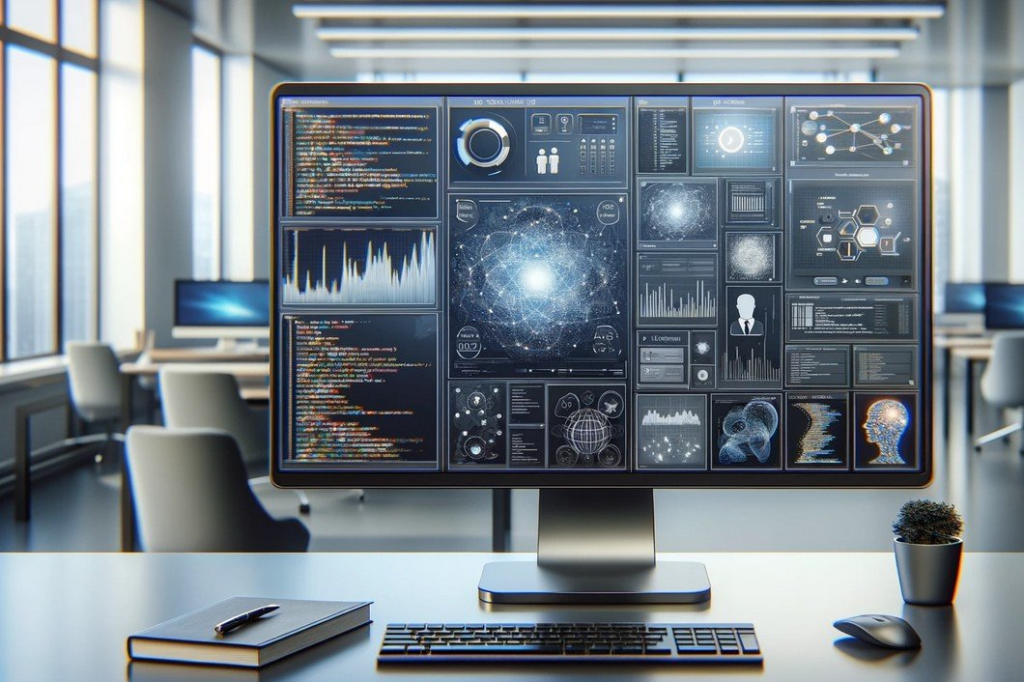
The software artificial intelligence (AI) industry is experiencing rapid growth and transformation, significantly impacting various sectors and the global economy.
Market Growth and Projections
In 2024, the AI market is projected to generate approximately $214 billion in revenue, with expectations to reach $1,339 billion by 2030, reflecting a compound annual growth rate (CAGR) of 36.6%.
Adoption Across Industries
AI integration is expanding across multiple sectors:
- Healthcare: AI enhances diagnostics, personalized medicine, and operational efficiency.
- Finance: AI-driven algorithms improve fraud detection, risk assessment, and customer service.
- Retail: AI enables personalized shopping experiences and optimizes supply chain management.
- Manufacturing: AI facilitates predictive maintenance and quality control.
Technological Advancements: The development of large language models (LLMs) and generative AI has revolutionized software capabilities, enabling more sophisticated and human-like interactions. Companies like OpenAI have pioneered these advancements, leading to widespread adoption and integration into various applications.
Investment Trends
Major technology companies are investing heavily in AI infrastructure. In 2024, tech giants such as Amazon, Microsoft, and Alphabet increased their AI data center infrastructure spending by 57%, totaling $133 billion. This surge underscores the strategic importance of AI in their business models.
Challenges and Considerations
Despite rapid advancements, the industry faces challenges, including:
- Data Limitations: The availability of quality training data is becoming a bottleneck, potentially slowing AI model development. Reuters
- Ethical Concerns: Issues related to bias, privacy, and the ethical use of AI are increasingly prominent.
- Regulatory Scrutiny: As AI systems become more integrated into daily life, they attract greater attention from regulators, especially concerning antitrust implications. Financial Times
Future Outlook
The AI software industry is poised for continued growth, with expectations of significant economic contributions. AI is projected to result in a 21% net increase in the United States GDP by 2030.
Forbes Ongoing research and innovation are likely to drive further integration of AI into various sectors, enhancing efficiency and creating new opportunities.
Disclaimer: This analysis is for informational purposes only and should not be considered financial advice. Consult with a financial advisor before making investment decisions.
Sectors Likely to Benefit Most from AI Integration
- Healthcare:
- AI Applications: Diagnostics, personalized medicine, drug discovery, operational efficiency, and patient care automation.
- Investment Opportunities: Companies developing AI-driven healthcare solutions, such as bioinformatics platforms, medical imaging tools, and telemedicine services.
- Finance:
- AI Applications: Fraud detection, risk management, automated trading systems, and customer service chatbots.
- Investment Opportunities: Financial institutions adopting AI for risk analysis, fintech companies leveraging AI, and AI service providers for the finance industry.
- Retail:
- AI Applications: Personalized shopping experiences, inventory optimization, supply chain efficiency, and demand forecasting.
- Investment Opportunities: Retailers using AI-powered tools, e-commerce platforms, and AI providers for retail analytics.
- Manufacturing and Logistics:
- AI Applications: Predictive maintenance, production optimization, robotic process automation (RPA), and autonomous vehicles.
- Investment Opportunities: Companies investing in smart factories, AI-driven supply chain management, and robotics firms.
- Technology and Software Development:
- AI Applications: Advanced AI tools, generative AI models, and SaaS platforms integrated with machine learning.
- Investment Opportunities: Leading AI research firms, cloud computing providers, and software companies focused on AI-powered solutions.
- Energy and Utilities:
- AI Applications: Optimizing energy production, predictive grid maintenance, and renewable energy forecasting.
- Investment Opportunities: Renewable energy companies, AI tools for grid management, and energy efficiency technology providers.
Strategic Positioning for Investors
- Diversified AI ETFs and Funds:
- Invest in exchange-traded funds (ETFs) or mutual funds that track the AI industry, providing exposure to a broad range of companies benefiting from AI integration.
- Focus on Infrastructure Providers:
- Look into semiconductor companies like NVIDIA and AMD, which provide the computing power essential for AI development and deployment.
- Cloud service providers like AWS, Azure, and Google Cloud, which host AI applications, are also key players.
- Early-Stage AI Innovators:
- Identify startups and early-stage companies with groundbreaking AI solutions in specific sectors, keeping an eye on venture capital or IPO opportunities.
- Sector-Specific Leaders:
- Target market leaders within high-growth sectors, such as AI-powered healthcare platforms or autonomous vehicle manufacturers.
- Monitor Emerging Trends:
- Stay informed about AI advancements, such as generative AI, edge computing, and AI ethics, to spot new investment opportunities as they arise.
- Evaluate Long-Term Growth Potential:
- Prioritize companies with strong research and development (R&D) capabilities, a robust AI product pipeline, and diversified applications across industries.
By aligning investments with sectors poised for AI-driven growth, investors can capitalize on the transformative potential of artificial intelligence over the next five years.
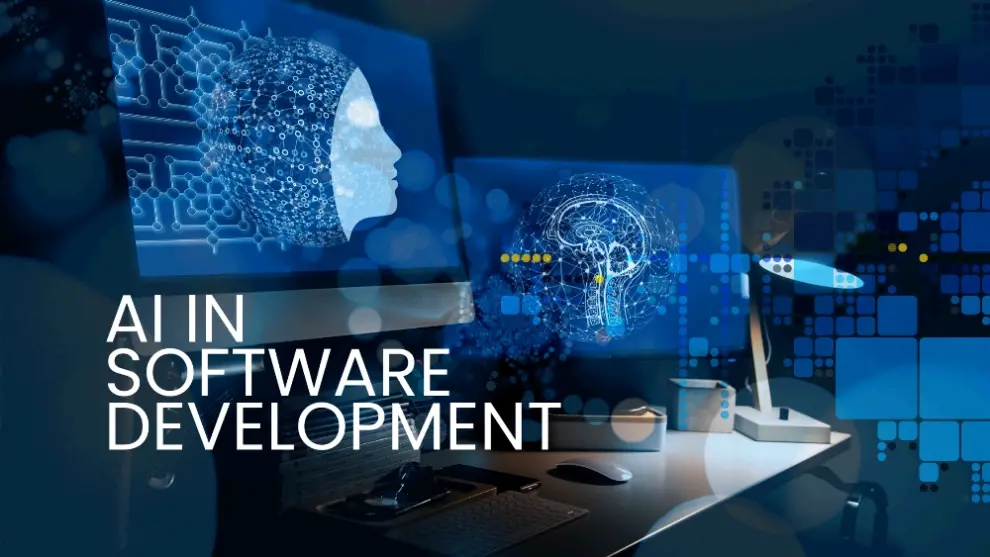
Impact of Generative AI and Large Language Models on the Competitive Landscape
- Transforming Industries:
- Content Creation and Media: Generative AI is revolutionizing the production of text, images, video, and music, enabling faster, cheaper, and more customized content creation.
- Customer Interaction: Large language models (LLMs) enhance conversational AI, driving better customer service, personalized experiences, and more efficient communication.
- Software Development: AI tools powered by LLMs automate code generation, debugging, and system optimization, boosting developer productivity.
- Healthcare and Life Sciences: LLMs assist in analyzing complex datasets, improving drug discovery, and personalizing medical care.
- Redefining Competitive Dynamics:
- Lower Barriers to Entry: Generative AI reduces the cost and time required to develop sophisticated applications, enabling startups and smaller firms to compete with established players.
- Ecosystem Expansion: Companies integrating LLMs into their products are creating new ecosystems around AI-driven applications, increasing user engagement and market share.
- Monetization Opportunities: Generative AI unlocks new revenue streams, such as subscription models, API monetization, and custom AI solutions for enterprises.
- Accelerated Innovation:
- Intense competition among tech giants is spurring rapid advancements in model performance, scalability, and application diversity, benefiting end-users across industries.
Companies Best Positioned to Lead in Generative AI and LLMs
- Technology Giants:
- OpenAI (Microsoft): Through its partnership with Microsoft, OpenAI’s GPT models power products like ChatGPT and are integrated into Microsoft Azure, offering cutting-edge generative AI solutions.
- Google (Alphabet): Google’s Bard and its DeepMind division leverage vast datasets and computational power to create advanced LLMs for search, cloud services, and more.
- Amazon: AWS offers AI tools like CodeWhisperer and Bedrock, enabling customers to build and deploy generative AI applications at scale.
- Specialized AI Companies:
- Anthropic: Focused on ethical AI development, Anthropic has gained attention for its safety-first approach to LLMs.
- Hugging Face: A key player in open-source AI, Hugging Face provides accessible AI models and frameworks, fostering innovation across industries.
- Stability AI: Known for its work in generative image models like Stable Diffusion, Stability AI drives innovation in visual content generation.
- Semiconductor Leaders:
- NVIDIA: As the leading provider of GPUs essential for training LLMs, NVIDIA is at the forefront of the AI hardware market.
- AMD: Competes with NVIDIA in providing high-performance hardware for AI workloads, benefiting from the generative AI boom.
- Emerging Startups:
- Companies like Cohere, Character.ai, and Jasper AI are developing niche applications for generative AI, targeting specific markets such as marketing, conversational AI, and creative industries.
Strategic Implications for Investors
- Invest in AI Infrastructure: Companies providing hardware (e.g., NVIDIA) or cloud platforms (e.g., AWS, Azure) are critical enablers of generative AI.
- Support Ecosystem Innovators: Firms building tools and frameworks for LLM integration, such as Hugging Face, are positioned for long-term growth.
- Diversify Across Applications: Explore opportunities in companies applying generative AI to specialized use cases, including healthcare, media, and finance.
Advancements in generative AI and LLMs are reshaping industries, creating a competitive landscape where companies with innovative solutions, scalable infrastructure, and ethical AI practices are likely to emerge as leaders.
Strategies for Mitigating Risks Related to Regulatory Scrutiny and Ethical Challenges in the AI Industry
- Focus on Companies with Transparent Practices:
- What to Look For: Companies that emphasize ethical AI development, prioritize data privacy, and adhere to transparent governance frameworks.
- Why It Matters: Transparent practices reduce the risk of regulatory penalties and help maintain public trust.
- Example: Companies like Microsoft and Google, which have published AI ethics guidelines and committed to responsible AI development.
- Diversify Across Regions and Sectors:
- What to Do: Invest in AI companies operating in regions with clear and stable regulatory frameworks.
- Why It Matters: Diversification reduces the impact of localized regulatory risks and spreads exposure across industries with varying levels of scrutiny.
- Example: AI applications in healthcare or renewable energy may face less regulatory risk compared to applications in finance or facial recognition.
- Monitor Regulatory Trends:
- What to Watch: Stay informed about emerging AI regulations, including laws addressing data privacy (e.g., GDPR), algorithmic accountability, and antitrust scrutiny.
- Why It Matters: Awareness of regulatory developments helps investors anticipate potential challenges and adjust portfolios proactively.
- Prioritize Companies Investing in Compliance:
- What to Look For: Firms dedicating resources to regulatory compliance, such as hiring legal and ethical AI experts or forming compliance committees.
- Why It Matters: Companies with robust compliance programs are better prepared to navigate regulatory changes, reducing legal and financial risks.
- Example: IBM has established AI-specific governance frameworks to ensure compliance with global regulations.
- Support Companies with Strong Ethical Standards:
- What to Look For: Firms that prioritize inclusivity, mitigate bias in AI systems, and implement safeguards against misuse of AI technologies.
- Why It Matters: Ethical AI development reduces the risk of reputational damage and regulatory crackdowns.
- Example: OpenAI has emphasized AI safety and collaboration with policymakers to ensure responsible deployment.
- Invest in Diverse AI Ecosystems:
- What to Do: Spread investments across AI infrastructure providers (e.g., cloud services, semiconductors) and application developers, reducing reliance on companies directly impacted by end-user regulations.
- Why It Matters: Diversification within the AI value chain mitigates sector-specific risks.
- Engage in Active Ownership:
- What to Do: For institutional investors, engage with company management to promote responsible AI practices and encourage proactive regulatory compliance.
- Why It Matters: Active ownership can influence companies to adopt stronger ethical and governance standards.
- Evaluate Risk Adjusted Returns:
- What to Do: Consider the risk-reward balance for AI investments, especially in high-risk applications like facial recognition or autonomous systems.
- Why It Matters: This ensures a cautious approach to high-growth but high-risk areas of AI.
- Leverage AI-Focused ETFs:
- What to Do: Consider AI-focused ETFs that diversify risk across a portfolio of companies, some of which may have lower regulatory exposure.
- Why It Matters: ETFs reduce individual stock risk while providing exposure to the broader AI industry.
- Stay Updated on Ethical AI Standards:
- What to Watch: Developments in AI governance frameworks from organizations like the OECD, EU AI Act, or U.S. FTC.
- Why It Matters: Early awareness of global standards helps investors align with companies adhering to leading practices.
By adopting these strategies, investors can mitigate the risks associated with regulatory scrutiny and ethical challenges while positioning themselves to capitalize on the long-term growth potential of the AI industry.
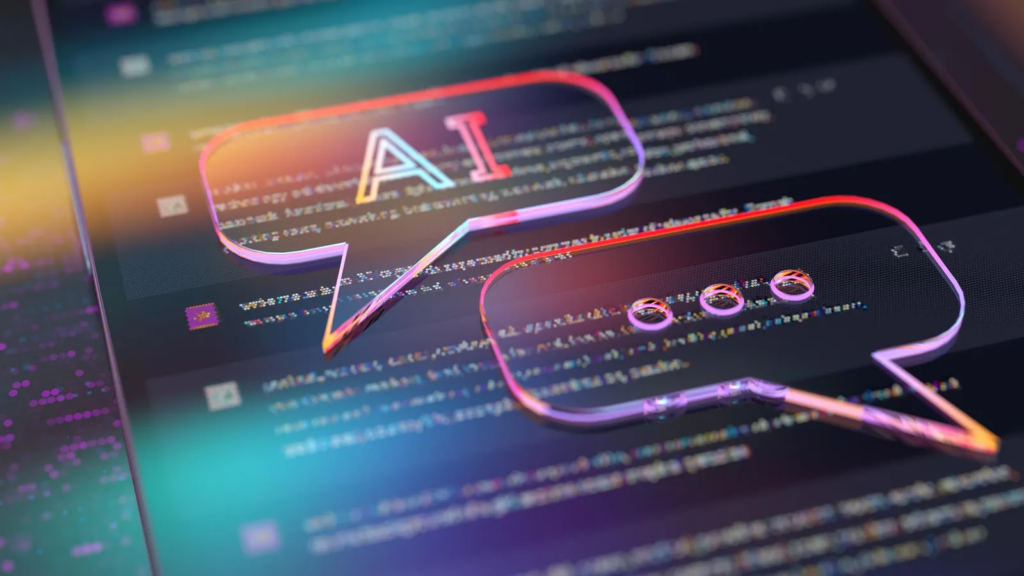
How Individual Investors Can Assess Opportunities in AI Infrastructure Supporting Industries
The rapid growth of AI and the increasing investments by tech giants in AI infrastructure present numerous opportunities for individual investors, particularly in industries like semiconductor manufacturing and cloud computing. Here’s how to evaluate and capitalize on these opportunities:
1. Focus on Key Players in Semiconductor Manufacturing
- Role of Semiconductors: Semiconductors are essential for powering AI applications, particularly GPUs (Graphics Processing Units), TPUs (Tensor Processing Units), and specialized AI chips.
- What to Look For:
- Companies with strong R&D in AI chip design (e.g., NVIDIA, AMD, Intel).
- Firms expanding production capacity to meet growing AI demand.
- Suppliers of advanced materials and equipment for chip manufacturing, such as ASML and TSMC.
- Evaluation Metrics:
- Revenue growth from AI-related segments.
- Competitive edge in chip performance and energy efficiency.
- Partnerships with tech giants for AI deployments.
- Example Opportunities: NVIDIA dominates the AI GPU market, while TSMC leads in advanced semiconductor fabrication.
2. Analyze Cloud Computing Providers
- Importance of Cloud Infrastructure: Cloud platforms like Amazon Web Services (AWS), Microsoft Azure, and Google Cloud underpin AI deployments by providing scalable, on-demand compute power.
- What to Look For:
- Market share and revenue growth in cloud services.
- AI-specific offerings such as pre-trained models, AI development frameworks, and cloud-based GPUs.
- Collaboration with enterprises for AI integrations.
- Evaluation Metrics:
- Growth in cloud services revenue.
- Number of enterprise clients adopting AI through cloud platforms.
- Investments in AI-optimized data centers.
- Example Opportunities: Microsoft Azure’s integration of OpenAI technologies or AWS’s Bedrock for generative AI solutions.
3. Diversify into Supporting Ecosystems
- Data Center Infrastructure: Companies providing hardware for data centers, including servers, cooling systems, and networking equipment (e.g., Arista Networks, Broadcom).
- Power and Energy Efficiency: Firms specializing in energy-efficient technologies for AI data centers, such as liquid cooling or renewable energy integration.
- What to Look For:
- Firms benefiting from increased demand for AI infrastructure upgrades.
- Companies at the forefront of sustainable and efficient energy solutions for data centers.
4. Monitor Emerging Technologies
- Quantum Computing: Quantum advancements may complement traditional AI hardware in the future.
- Edge Computing: Companies focusing on edge devices and on-premise AI solutions.
- What to Watch:
- Firms innovating in areas like edge AI chips (e.g., Qualcomm).
- Partnerships between hardware companies and tech giants exploring next-gen AI solutions.
5. Assess Market Trends and Risks
- AI Adoption Growth: Track industries adopting AI at scale (e.g., healthcare, automotive, and retail) to gauge demand for supporting infrastructure.
- Supply Chain Stability: Evaluate companies with resilient supply chains, especially in semiconductors, which can face disruptions.
- Geopolitical Risks: Monitor trade policies and international relations, particularly concerning semiconductor manufacturing regions like Taiwan.
6. Leverage Investment Vehicles
- AI-Focused ETFs: ETFs such as iShares Robotics and AI ETF (IRBO) or VanEck Semiconductor ETF (SMH) provide diversified exposure to AI infrastructure.
- Cloud Computing ETFs: Funds like First Trust Cloud Computing ETF (SKYY) offer access to cloud-based investments.
7. Monitor Financial Indicators
- Revenue growth from AI-related products.
- Capital expenditures on expanding AI-related infrastructure.
- Valuation metrics (P/E ratio, price-to-sales ratio) compared to industry averages.
By focusing on these strategies, individual investors can identify high-potential companies in semiconductor manufacturing, cloud computing, and other supporting industries poised to benefit from the AI infrastructure boom.
Metrics and Indicators to Monitor AI’s Economic Contributions and Disruptive Potential
To effectively evaluate the AI industry’s progress and its potential for economic disruption, investors should focus on key quantitative and qualitative metrics. Here are the critical areas to monitor:
1. Market Adoption and Growth Metrics
- Revenue Growth in AI Markets:
- Track the overall revenue generated by AI-related industries, including AI software, hardware, and services.
- Example: Projections showing AI market revenue reaching $1.34 trillion by 2030 (CAGR of 36.6%).
- Industry-Specific AI Adoption:
- Monitor adoption rates of AI in key sectors such as healthcare, finance, retail, and manufacturing.
- Key Insight: Faster adoption in sectors like healthcare and autonomous vehicles indicates disruptive potential.
2. Technological Advancements
- Performance of AI Models:
- Monitor advancements in generative AI, large language models (LLMs), and domain-specific AI applications.
- Example Metrics:
- Model size (parameters), processing speed, and cost efficiency.
- Patents and Research Papers:
- Track the number of patents filed or research papers published related to AI technologies, as these reflect innovation intensity.
3. Economic Contribution Metrics
- AI’s Share in Global GDP Growth:
- Evaluate the percentage of GDP growth attributed to AI technologies.
- Example: AI is projected to add 21% to U.S. GDP by 2030.
- Labor Productivity Gains:
- Assess improvements in productivity due to AI deployment, especially in sectors like manufacturing and logistics.
4. Investment and Funding Trends
- Venture Capital Funding:
- Track investments in AI startups and technology incubators.
- Key Metric: Amount of venture funding and the number of new AI-focused startups.
- Corporate AI Spending:
- Monitor tech giants’ expenditure on AI R&D, infrastructure, and acquisitions.
- Example: In 2024, cloud providers increased AI infrastructure spending by 57%.
5. Infrastructure and Ecosystem Metrics
- AI Hardware Development:
- Track advancements in semiconductor production and the deployment of GPUs and TPUs optimized for AI.
- Key Players: NVIDIA, AMD, TSMC.
- Cloud AI Usage:
- Monitor adoption rates of AI-as-a-Service (AIaaS) platforms provided by AWS, Azure, and Google Cloud.
6. Regulation and Ethical Compliance
- AI Governance Metrics:
- Assess compliance with new regulatory frameworks like the EU AI Act or U.S. FTC guidelines.
- Monitor the impact of regulations on innovation and deployment timelines.
- Ethical AI Metrics:
- Track companies’ investments in bias reduction, privacy safeguards, and ethical AI initiatives.
7. Labor Market Impact
- Job Creation and Displacement:
- Measure the net effect of AI on job markets, including jobs created in AI-related fields versus those displaced by automation.
- Skills Gap Analysis:
- Assess trends in workforce upskilling and demand for AI-specific skillsets.
8. Competitive Landscape Indicators
- Market Share of AI Leaders:
- Monitor the competitive position of major AI companies like OpenAI (Microsoft), Google, and NVIDIA.
- Mergers and Acquisitions:
- Evaluate consolidation trends as companies acquire AI startups or expand capabilities.
9. End-User Metrics
- Consumer Adoption Rates:
- Measure the percentage of consumers using AI-enabled tools or services.
- Enterprise AI Integration:
- Assess the proportion of enterprises adopting AI for operations, customer engagement, and decision-making.
10. Macro-Level Indicators
- AI Contribution to Exports:
- Track the export value of AI technologies and solutions from leading economies.
- Geopolitical Trends:
- Monitor how AI capabilities affect global economic competition, particularly between the U.S. and China.
Conclusion
Investors can use these metrics to track the AI industry’s progress and evaluate its potential to disrupt traditional industries. By staying informed on technological advancements, economic contributions, and regulatory trends, they can make well-informed decisions to capitalize on AI-driven growth.